[Published online Journal of Computer Chemistry, Japan Vol.19, 57-63, by J-STAGE] <Title:> Antiferromagnetic Orderings of Alkali-metal Nanoclusters Arrayed in Sodalite Crystal Studied by μSR and Other Microscopic Probes
<Author(s):> Takehito NAKANO
<Corresponding author E-Mill:> takehito.nakano.phys(at)vc.ibaraki.ac.jp
<Abstract:> Abstract: Alkali metal clusters with an unpaired electron can be periodically arranged in a body-centered cubic structure in sodalite, a type of aluminosilicate zeolite, to form a Mott insulator accompanied with an antiferromagnetic ordering. This system does not contain any magnetic elements and is a novel magnetic system in which the magnetic order is realized by alkali metal s-electrons. In order to investigate the origin of the s-electron magnetism in detail, we present examples of studies using muon spin rotation/relaxation (μSR), synchrotron radiation M ssbauer spectroscopy, and neutron diffraction techniques. The spatial expansion of the s-electron wave functions of the nanoclusters with increasing alkali metal content has been directly observed by these experimental methods. This enhances the exchange interaction and increases the transition temperature (N el temperature). A very simple model material of the Mott-Hubbard system is realized in s-electrons. We also point out that there are great expectations for the future contribution of computer science to this material system, especially to μSR experiments.
<Keywords:> Alkali-metal cluster, Zeolite, Sodalite, Antiferromagnetism, Mott insulator, Muon spin rotation/relaxation, Synchrotron radiation M ssbauer spectroscopy, Neutron diffraction
<URL:> https://www.jstage.jst.go.jp/article/jccj/19/3/19_2020-0020/_article/-char/ja/
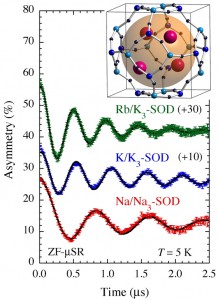